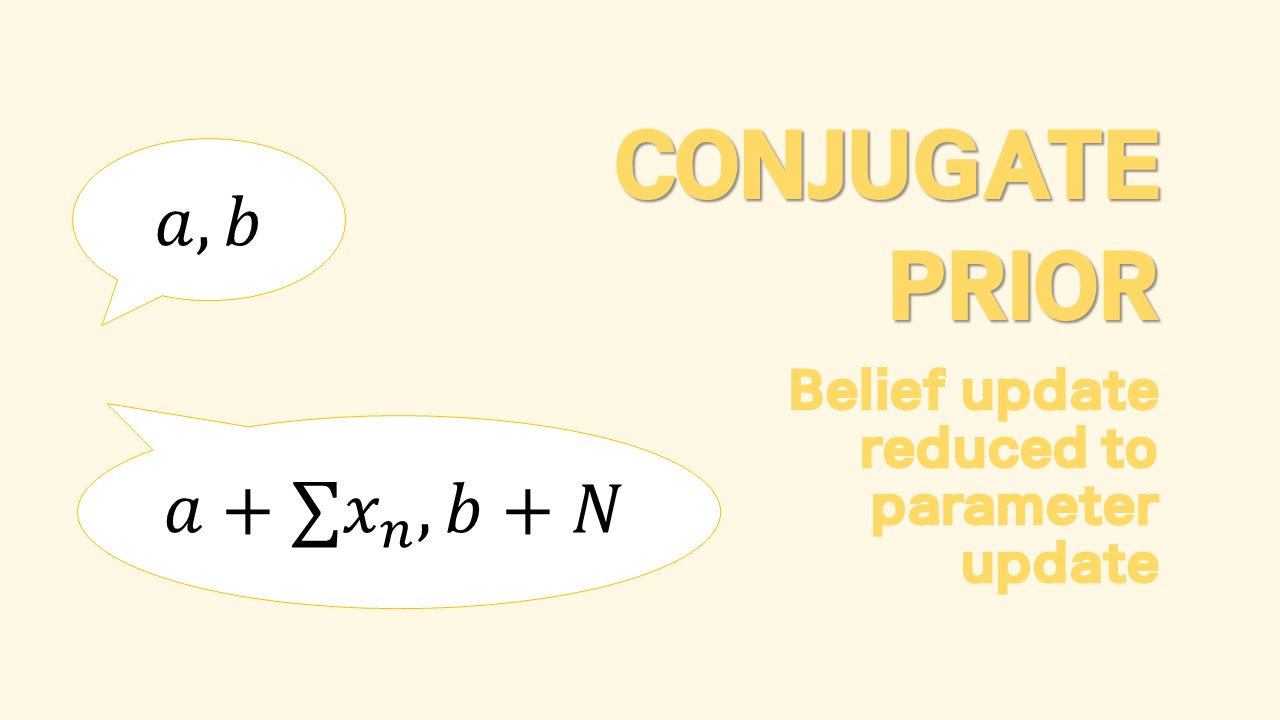
Conjugate Prior for Univariate - Poisson Model
library(ggplot2) library(cowplot) library(reshape) Bayesian Update and Prediction Given a data $\mathbf{D}={x_1, x_2, …, x_n}$, once a likelihood model $p(\mathbf{D}|\theta)$ and a prior on a parameter $p(\theta)$ are specified, Bayesian inference produces an updated belief on $\theta$.
$$ \begin{align} \text{Prior Belief}&\quad p(\theta)\\\
\text{Likelihood}&\quad p(\mathbf{D}|\theta)\\\
\text{Updated (Posterior)}&\quad p(\theta|\mathbf{D}) = \dfrac{p(\mathbf{D}|\theta)p(\theta)}{\int p(\mathbf{D}|\theta)p(\theta)d\theta} \propto p(\mathbf{D}|\theta)p(\theta) \end{align} $$
Our interest may extend to the prediction the new value $\tilde{x}$ that would be generated from the same sampling distribution.