Variational Inference and Bayesian Gaussian Mixture Model
When your computer cannot handle the burden of MCMC, you might as well allow for some bias and do some heavy math yourself
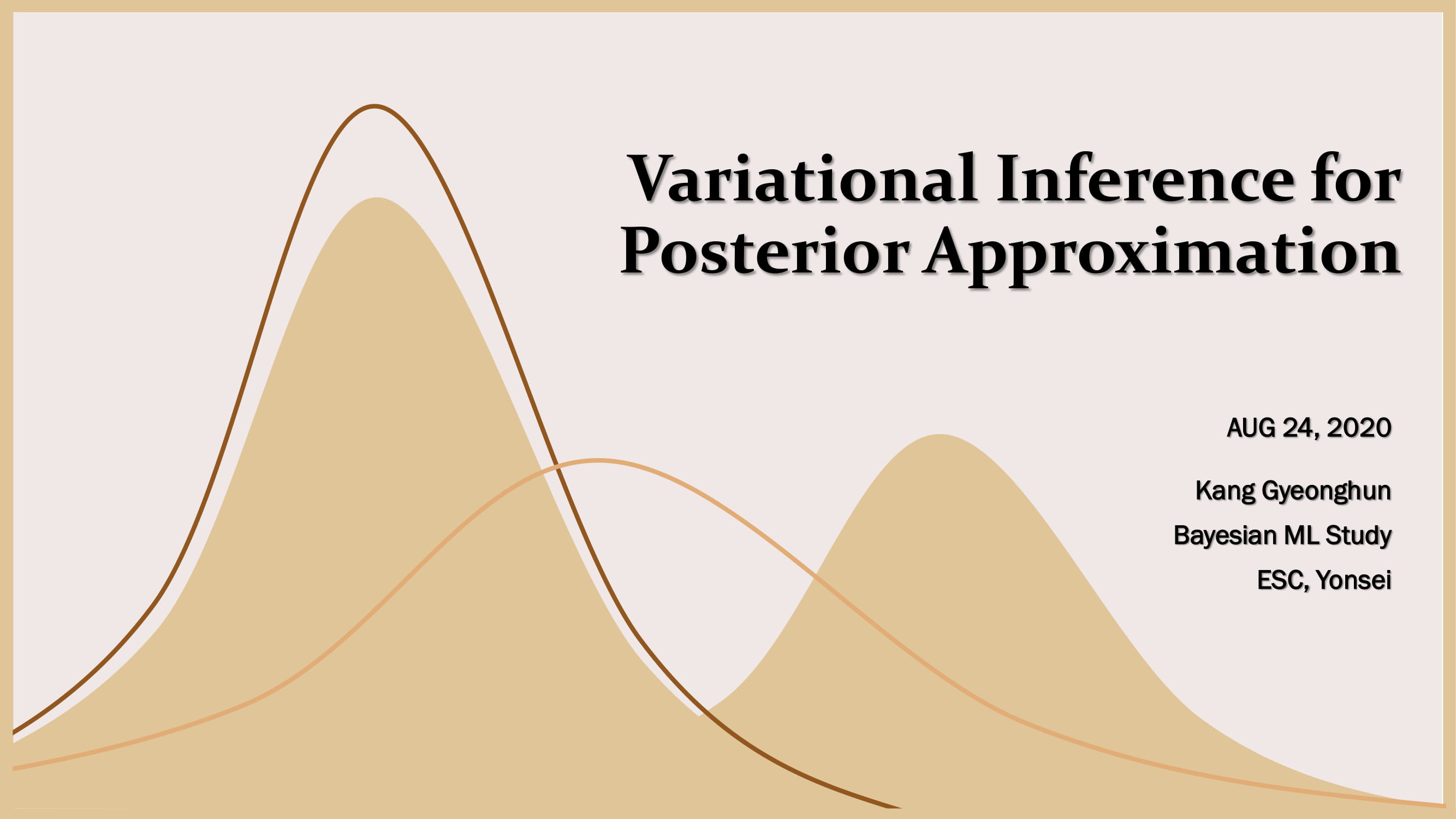
When your computer cannot handle the burden of MCMC, you might as well allow for some bias and do some heavy math yourself